Generative AI: The Promise, the Peril
The Problem: The More the Jargon We Use, The Less We Know!
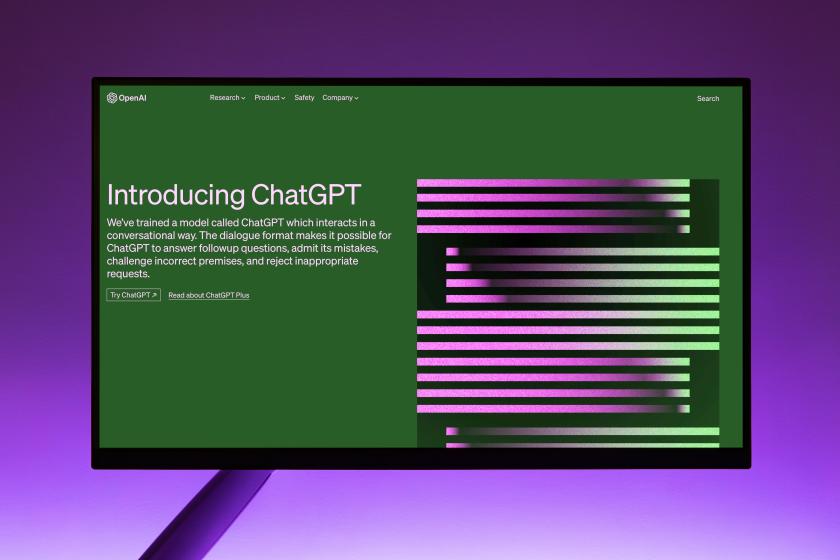
The train—oops, the bullet train—arrived on November 30, 2022. On this date, OpenAI—a nonprofit organization—introduced ChatGPT, which is (simply stated) a computer program (available through an app and a website) that allows its user to instruct (via text or voice) another program (called GPT, which means generative pre-trained transformer) to generate an output based on historical (and not real-time) information on the internet. Between November 30, 2022, and October 31, 2023, chat.openai.com received 14.75 billion visits. Interestingly, nearly 75% of these visits are direct through the app or by entering the website link in a web browser. These numbers are unprecedented!
Countless individuals and organizations have claimed expertise on not only AI but also a host of its sibling terms, such as gen AI (generative artificial intelligence), large language model (LLM), and artificial general intelligence (AGI). The reactions have varied from surprise (e.g., how cool!) to fear (e.g., my advertising agency will run out of business!) to excitement (e.g., how do I use it to cut costs and boost employee productivity)! Appropriating these reactions, multiple people have edited their LinkedIn titles to include AI/ML, LLM, and whatnot, and many AI consulting companies have popped up. Scratch the surface, and you realize that individuals and organizations lack a true understanding of generative AI.
The more this jargon builds, the harder it is for a person to ask fundamental questions, such as what organizational/business problems—if any—can generative AI solve. Equally importantly, what new problems can it create? The latter question is important because if people believe that generative AI can eliminate their jobs, they will not use it (remember what happened in Hollywood? Humans 1, AI 0). Thus, the questions are not only about the technology’s ability but also people’s willingness to try it. Between ability and willingness, an opportunity awaits!
The Solution for Senior Managers
Senior managers who are accountable for profit and loss should begin the conversation with one (and I emphasize one because the start must be with one) organizational problem, the current solution(s), and whether—and if yes, how and why—generative AI can solve the problem more effectively and/or more efficiently solution. It is also important to ask whether/how/why generative AI aggravates the problem.
A disclaimer is in order. Because generative AI is still in the infancy stage, I doubt anyone can produce evidence on how the use of generative AI will boost effectiveness and/or efficiency. Further, the evidence—even if available—may not apply to the focal organization. Therefore, when an organization considers generative AI, it must commit to a pilot project, evaluate the outcomes, and decide whether to expand the application of generative AI. Therefore, what is needed is a consultant who works with the organization to measure whether, how, and why the use of generative AI produced (or did not produce) the anticipated outcomes. Such a methodological approach will prevent speculation and help managers know what they must do and not do.
The Solution for Technical Staff
Technical staff needs to use a generative AI tool (such as ChatGPT-4, Ernie) and not merely talk about generative AI. For example, using ChatGPT-4 means paying OpenAI $20 for a month and using it in various ways that align with either the user’s personal interests or their organization’s operations.
For example, I have to decide whether to set up a fulfillment center in Ontario or Quebec. List the pros and cons for each province.
How much money would I save each month if all my customers used a chatbot built on GPT-4 for the first line of phone support?
Feed ChatGPT-4 a data file that has anonymized data on your customers and ask it to measure each customer on their lifetime value. Ask it to identify customers to whom you should send a 20% discount coupon.
Enter the world of creativity! Ask ChatGPT-4 to design a product, come up with a brand name, create a print advertisement, and compose lyrics or music for a birthday song.
The broader aim is to explore the limits of ChatGPT-4 and know—based on YOUR experience and not some guru’s views—what value, if any, ChatGPT offers you and/or your organization.
Now, go and talk and experience the bounce in your voice. Nothing beats the confidence one gains from learning by doing.